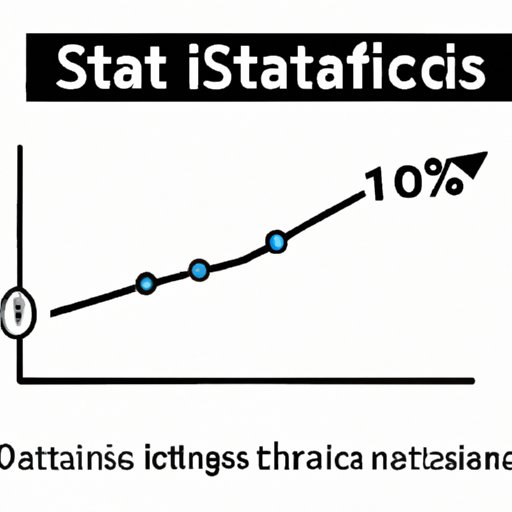
Introduction
Statistics have become an indispensable tool for making sense of the world around us. They help us predict elections, understand consumer behavior, and make informed business decisions. However, statistics are also frequently manipulated, distorted and presented to deceive the public. Such deception is a concern to all of us as it can adversely affect our judgments and decisions. In this article, we will explore how to spot and avoid being deceived by misleading statistics.
Manipulating Data for Desired Outcome
Data manipulation refers to the process of selectively using, interpreting or presenting data to achieve a desired result. One way this can be accomplished is by controlling sample sizes. In other words, the smaller the sample size, the greater the chance of obtaining data that appears to support a particular position. Additionally, using biased questions in survey research can skew the results. Selectively including or excluding outliers, or cherry-picking specific data points can also distort the representation of data.
Examples of past manipulations include political polls touting a head-to-head lead for a political candidate, advertisements boasting unparalleled product success, and scientific studies with selective data representation.
Common Statistical Fallacies
Correlation vs. causation is a common fallacy. Merely because two variables are correlated in time does not mean their relationship implies a causation between them. This fallacy can be used to suggest that causing A will produce B.
Anecdotal evidence is another such fallacy, where people believe that because they know someone who was affected by something, it must be a general occurrence among the population. This fallacy can be used on a grand scale by projecting one individual’s experience onto an entire population.
Averages vs. distributions is another misrepresentation of data where the sample is used to represent the whole population. For instance, the average income may be commonly used to measure wealth in a population, but taking into account income distribution is more accurate as the income deviation from average tells a different story.
Overgeneralization is also a common fallacy, where believing something is true for an entire population because it is true for a subset thereof. This overgeneralization is often used in professed scientific results.
Distorting Graphs and Charts
Graphs and charts are pictures of data that can be also manipulated to mislead. Graphs with disproportionate scales or cherry-picked data points can misrepresent true relationships or reveal false ones. Changing scales can eliminate key information: for example, if a graph that reports unemployment rates only goes from ten percent to twelve percent, any drop to nine percent will appear as a huge drop on the chart.
Truncated axes are another way of distorting data which elides information or obscures substantial changes, which can provide an inaccurate picture. Misleading graphs and charts can include comparisons of pie sizes, the use of 3D charts that exaggerate volumes, and so forth.
Case Study of Deceptive Statistics
The 2018 World Cup quarter-final football match between England and Sweden is an example of deceptive statistics. Despite the fact that Sweden possessed the ball 56% of the time, passed the ball more accurately, and had lower fouls, the score favored England. These statistics are deceptive because of England’s very high performance rate in opportunities. England took four shot opportunities, and of those, three scored, and one missed. That is 75% shot-success rate.
Importance of Context in Interpreting Statistics
Context is crucial to interpreting statistical significance. Failure to include the broader context can create misleading data or ideas. For instance, crime statistics could be used selectively to create an image for a particular area that is not representative of reality. Economic indicators applied can be misleading: The poverty rate indicator used by the United States government doesn’t take into account various factors like fair market rent, cost of living, or changing economic situations like inflation, leading to errors in the intended pop culture understanding of the issue.
Spotting and Avoiding Misleading Statistics
Readers ought to take even well-sourced claims with a grain of salt. Check sources, question methodology, confirm with reputable alternative reporting, examine research, and ask questions. Additionally, understand the context surrounding the data and have a sense for common triggers for deception. All it takes is an alert, prismatic view of the data to know whether it is true.
Ethical Implications of Deceptive Statistics
The ethical implications of deceptive statistics are vast. When statistics have played a role in something as important as healthcare or politics, deception can result in the loss of public trust in physicians, professionals, or politicians. The damage can be extensive, including bad decision-making, cultural chaos, and wasted funds. Ethical implications, therefore, are severe and should be taken seriously.
Conclusion
Statistics are an integral part of our world and have enabled us to create progress in countless areas. Unfortunately, lying with statistics is rampant and can negatively affect everyone. It’s essential to understand the various ways in which data can mislead and how to avoid being deceived. By being aware, critical, and always questioning what we see, we can gain a better understanding of the world around us and make more informed decisions.